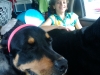
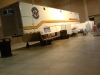
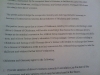
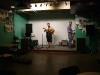
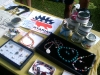
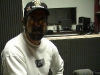
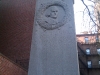
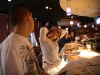
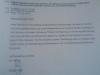
This is part of the i2 solution. It’s pretty scary how it spiders the web looking for usage patterns to determine who MIGHT be a criminal.
Authorship Analysis: Authorship Analysis Authorship analysis attempts to determine the likelihood of a particular author having written a piece of work based on some characteristics of the author [2]. The essence of this technique, is the formation of a set of metrics, or forensics, that remain relatively constant for a large number of writings created by the same person [3]. In cyber crime research context, this technique can help determine whether a set of illegal Internet messages belong to the same user based on the person’s writing style. [2] A. Gray, P. Sallis, and S. MacDonell, “Software forensics: Extending authorship analysis techniques to computer programs,” in Proc. 3rd Biannual Conf. Int. Assoc. of Forensic Linguists (IAFL’97), pages 1-8, 1997. [3] O. de Vel, “Mining e-mail authorship,” in Proc. Workshop on Text Mining, ACM International Conference on Knowledge Discovery and Data Mining (KDD) 2000.
Experiment – Data Collection: Experiment – Data Collection An experiment was conducted to test the prediction accuracy of authorship analysis algorithm. 2 types of data were used: 70 email messages 3 students provided 20-30 email messages each. Messages were randomly chosen by their authors and covered a variety of topics. 153 newsgroup messages 3 popular USENET newsgroups related to software trading were selected. misc.forsale.computers.other.software misc.forsale.computers.pc-specific.software misc.forsale.computers.mac-specific.software 9 users who frequently posted messages in the 3 newsgroups were chosen. Messages posted by these users were manually checked, with the help from domain experts, to determine whether they were illegal (i.e. involving sales of pirate software). 10-30 messages per user were manually downloaded that contained illegal content.
Experiment – Feature Extraction: Experiment – Feature Extraction Previous research suggested that style markers and structural features are good indicators of an author’s style [3]. Three types of message text features were used in this experiment to determine the authorship: Style Markers (205 features) average sentence length, total number of characters, total number of punctuations, etc. Structural Features (11 features) has a greeting, has a salutation, position of reply text, number of attachments, etc. Content-specific Features (9 features, for newsgroup messages only) has a list of products, position of price (in subject, in body, in list), etc. Style markers were extracted automatically using programs. Structural and content-specific features were extracted manually. [3] O. de Vel, “Mining e-mail authorship,” in Proc. Workshop on Text Mining, ACM International Conference on Knowledge Discovery and Data Mining (KDD) 2000.
Experiment – Classification Results: Experiment – Classification Results A Support Vector Machine classifier [4] was used to predict the authorship of the messages based on the extracted features. 10-fold cross validation method was used. Improvement in accuracy was observed with different combinations of message features. [4] C.-W. Hsu and C.-J. Lin. “A comparison on methods for multi-class support vector machines,” IEEE Transactions on Neural Networks, 13, pages 415-425, 2002.